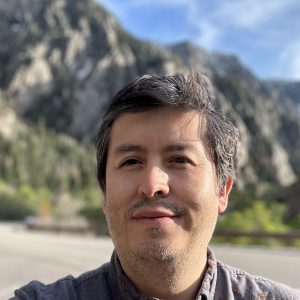
TUSCALOOSA, Ala. – A new study published in the Journal of Geophysical Research: Machine Learning and Computation has found that observed water flow in downstream rivers can be used to accurately estimate upstream flows, even in places without physical sensors. This breakthrough could help address one of hydrology’s most persistent challenges: estimating streamflow in ungauged basins.
The research team used a machine learning model known as long short-term memory to analyze synthetic watershed networks, showing that this “surrogate gauging” method significantly outperforms traditional parameter-transfer approaches in predicting streamflow across simulated basins. The model achieved better performance in approximately 89% of the synthetic basins tested, including under both low- and high-flow conditions.
“Most rivers in the U.S. and around the world don’t have flow gauges,” said University of Alabama Ph.D. student and lead researcher Andrés Ramírez Molina. “That’s a big problem for flood prediction, water management and emergency planning. Our approach offers a flexible and scalable way to fill those data gaps without needing to install new sensors everywhere.”
A Simple But Powerful Idea
At the core of the study is a straightforward concept: model any watershed as a pair of connected basins, with one upstream without a gauge and one downstream with observed data. By treating watersheds this way, researchers can train models to learn the upstream behavior based on what happens downstream, plus information about local terrain, weather and other physical features.
“It’s like listening to multiple people talk at once and using visual cues like lip movements to separate their voices,” described Ramírez Molina. “We’re using downstream flow as the signal and watershed characteristics as the visual context to estimate what’s happening upstream.”
Why It Matters
Reliable streamflow data is essential for managing water resources, issuing flood warnings, designing infrastructure and enforcing environmental regulations. However, most river segments, especially in rural or remote regions, are unmonitored. Without gauges, traditional models rely on assumptions or parameter transfers from other watersheds, which often fall short due to local differences in soil, land cover and precipitation.
This new method sidesteps these issues by incorporating observed data from downstream gauges to estimate upstream conditions, essentially acting as a surrogate gauge, and ultimately offering a more robust alternative to parameter transfer.
From Simulation to Real-world Rivers
The team began with synthetic watershed networks, which allowed them to control and observe all variables in the system. This helped them test the model’s capabilities without the limitations of sparse real-world data.
“The synthetic setup gave us full visibility,” said Ramírez Molina. “We could validate the model at every step. Now that we’ve demonstrated its potential in a controlled synthetic environment, the next step is to apply it to real basins with known upstream and downstream flows.” If successful in real-world testing, the approach could eventually scale to national or even global hydrologic models, helping forecasters, planners and water managers fill in the blanks where sensor networks fall short.