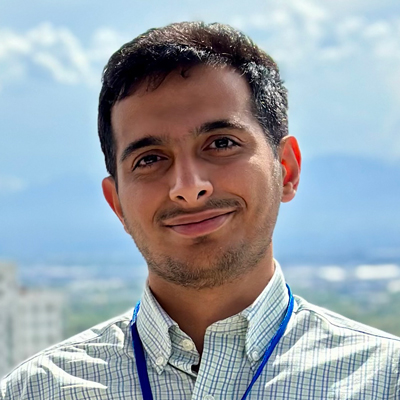
TUSCALOOSA, Ala. – A newly published study from University of Alabama Ph.D. student Savalan Naser Neisary and his advisor Steven Burian introduces a novel machine learning framework that dramatically improves the accuracy of the National Water Model (NWM) in predicting streamflow in complex, regulated basins and the drought-prone Western U.S.
Biases in NWM predictions undermine the reliability of streamflow forecasts, leading to misinformed water management decisions, especially in drought-prone and infrastructure-heavy regions.
The research, published in Environmental Modelling & Software, presents a model-agnostic post-processing machine learning approach that corrects biases in NWM predictions by incorporating key streamflow drivers such as upstream reservoir storage, snowpack data and catchment characteristics.
“In places like the Western U.S., where water systems are tightly managed and dependent on snowmelt, the National Water Model struggles—especially in low-flow and drought conditions,” said Neisary. “Our framework helps fix that.”
The team tested the framework in watersheds feeding the Great Salt Lake, a critical but shrinking water body in Utah. The results were striking:
- 65% improvement in prediction accuracy (Kling-Gupta Efficiency)
- 335% reduction in bias
- 225% improvement in low-flow predictions at reservoir-impacted sites
This breakthrough could significantly enhance the tools available to water managers, modelers and drought plannersacross the country, particularly as the NWM is poised to become a cornerstone of national water forecasting operations.
Crucially, the method does not require explicit modeling of infrastructure operations, making it flexible and scalable to other regions.
“This is like having an editor polish a rough draft,” Neisary explained. “The National Water Model does the initial writing, but the machine learning framework sharpens it to reflect the realities on the ground.”
As water forecasting becomes increasingly critical, this new machine learning framework offers a powerful, scalable way to boost the performance of the NWM without needing to explicitly model complex infrastructure. By significantly improving accuracy where it’s needed most, this research arms water managers and planners with sharper, more reliable tools to respond to drought, manage water supply and protect vulnerable water supplies across the Western U.S. and beyond.